Published: October 16, 2020
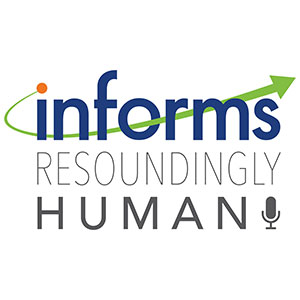
No one likes waiting in lines, it’s a fact. Whether at the grocery store checkout searching desperately for the shortest line, or watching the clock tick while waiting our turn at the dentist’s office, or at an amusement park, looking at the long lines and deciding how badly we really want to ride our favorite ride, most of will do everything we can to avoid waiting.
And even since the arrival of the coronavirus pandemic, when so many aspects of our lives are now conducted virtually, we still find ourselves waiting in lines, or in this case, in virtual queues, both online or by phone.
For this episode, I am joined by Qiuping Yu, professor with the Georgia Institute of Technology, to discuss her study “The Reference Effect of Delay Announcements: A Field Experiment,“ which will be published in an upcoming edition of the INFORMS journal Management Science. Her research explores our new normal of virtual waiting rooms and what companies can do to ensure excellent service and customer appreciation. The key comes in the form of carefully managed WTI, or wait time information.
Americans spend roughly 37 billion hours each year waiting in line. In fact, based on a recent survey it reported that wait related issues are the number one reason retailers lose customers. As queues go virtual, optimizing how you provide wait time estimates is an inexpensive yet very effective tool to significantly improve your customers’ experience for any business that’s thinking of implementing a virtual queue.
Interviewed this episode:
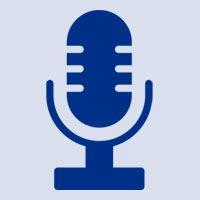
Qiuping Yu
Georgia Institute of Technology
Qiuping Yu is an assistant professor of Operations Management and Business Analytics at the Scheller College of Business, Georgia Institute of Technology. She conducts research on data-driven service operations with a focus on digital service design (e.g., design of virtual queues), workforce analytics (e.g., labor scheduling and fairness issues), and management of large-scale service networks. Besides applying various state-of-art empirical and analytical methods, she also develops frameworks that combine techniques from both casual inference and machine learning. Her research utilizes large and highly granular proprietary data from a variety of firms in the service and retail industries. She has worked with major ridesharing platforms, restaurants, call centers, freight matching platforms, and fashion retailers both in the US and in other countries.
Her research has been published in Management Science and Harvard Business Review and was given extensive coverage in media outlets including the Wall Street Journal, Bloomberg Businessweek, American Enterprise Institute, Foundation for Economic Education, the Cato Institute, the Tennessee Star, KCBS News Radio San Francisco, and INFORMS Resoundingly Human Podcast. Her research was recognized with multiple awards and grants. She teaches Revenue Management and Data Analytics for Business at Georgia Tech, and was recognized by the Student Recognition of Excellence in Teaching Award.
Want to learn more? Check out the additional resources and links listed below for more information about what was discussed in the episode.
“When providing wait times, it pays to underpromise and overdeliver,” Harvard Business Review