Published: November 20, 2019
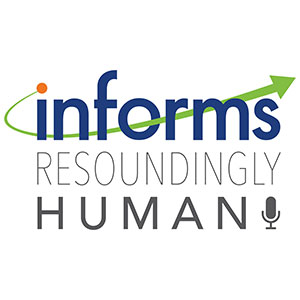
This episode is one of a special series recorded during the 2019 INFORMS Annual Meeting in Seattle. Joining me for this episode is Maryam Zokaei Nikoo from Pennsylvania State University to discuss research she shared at this year’s Annual Meeting. Her presentation, “Using Artificial Intelligence and Natural Language Processing to Predict Alzheimer’s Disease,” is based on research to improve early detection of Alzheimer’s, a disease currently impacting 5.8 million Americans and the 6th leading cause of death in the U.S.
Want to take a deeper dive? Check out the brief introduction of the research referenced in this podcast below for more information:
“Developing interpretable prediction models can build trust especially in the healthcare domain. In recent years, deep learning models have performed promisingly in various applications including computer vision, audio processing, and natural language processing. However, deep learning models are black-box models, which make their application difficult in healthcare due to lack of interpretability.
In this study, natural language processing (NLP) and hierarchical deep learning are combined to detect the onset of Alzheimer’s disease based on the longitudinal patient interview data, who were asked to describe a picture (Cookie-Theft). A novel three-level hierarchical recurrent neural network is designed with unique attention over self-attention (AoS) mechanism to develop a powerful and interpretable model that can explain the most important lexical memory-related patterns without the need for any feature engineering.
While proof of concept is given for Alzheimer’s disease, this type of modeling framework can also be employed to predict other degenerative neurological diseases.“
Interviewed this episode:
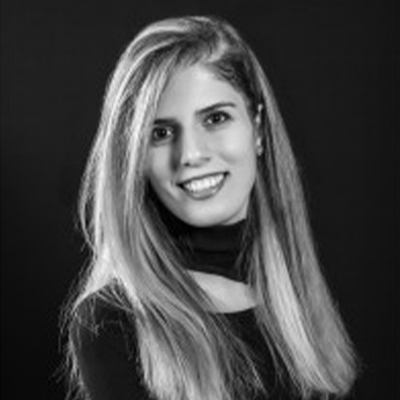
Maryam Zokaei Nikoo
Pennsylvania State University
Episode Transcript
Contact us to request transcript.