Published: April 3, 2025
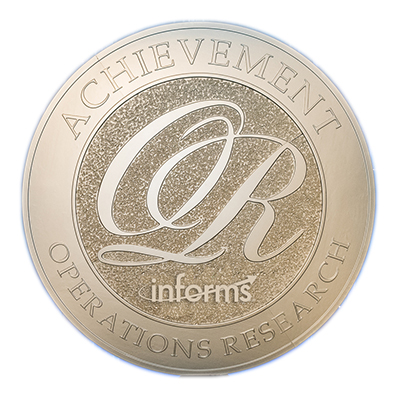
Welcome to the latest in our special series of Resoundingly Human podcasts highlighting the finalist teams for the 2025 Franz Edelman Award, the Nobel Prize of Analytics, which will be awarded at the upcoming 2025 INFORMS Analytics+ Conference in Indianapolis this April.
These finalist projects represent teams from around the world who have leveraged advanced analytics to transform their organizations, address their most significant challenges, and better serve their customers and communities. Today I’m joined by members of the team representing WM to discuss their finalist project in the leadup to the Franz Edelman competition.
The collaboration between our teams was really the differentiator in really making this work. At WM, we’ve actually tried this twice over the last 15 years, to use data and analytics to dynamically route our industrial line of business and we weren’t successful frankly. The collaboration of our teams coming together to dynamically optimize 22,000 customer services across 500 hauling sites and 4,000 routes a day was a herculean effort. If you think about getting all of the data right that has to happen, and has to be correct behind that, that’s no small task. So building an optimizer that can handle that much data and produce 4,000 routes in 45-minutes every night for tomorrow took a lot from our team, of the operations research team and data and analytics. Beyond that really getting our field team to trust the optimization, really working with them hand-in-hand to test the solution and let them see it before it went live is what really helped in that collaboration. To get all the field roles involved, even all the way down to our drivers, to get them involved, to get their feedback.
Interviewed this episode:
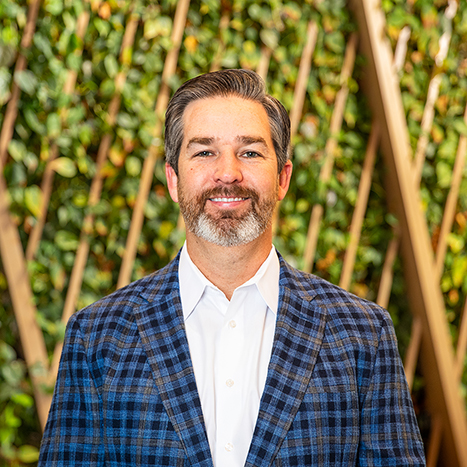
Marcel Dalby
WM
Marcel Dalby is the vice president of collections operations and business optimization at WM overseeing the optimization efforts across company’s collection operations, fleet, disposal, indusial automation, routing & logistics, and recycling operations. His team is responsible to drive cost savings, increase efficiencies and improve overall customer service through the implementation of innovative technologies and improved enterprise business processes. He previously led WM’s collection and landfill operations in Jacksonville, Florida with responsibility for area operations, sales, service, strategic planning, and financial performance. He holds a Bachelor of Business Administration Management degree from Texas State University.
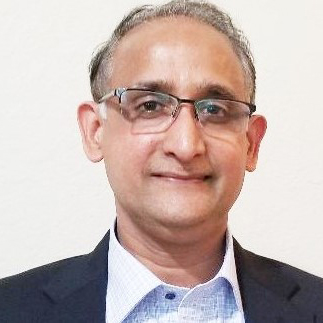
Hema Pillutla
WM
Hema Pillutla is the senior director of innovation and business optimization at WM leading the operations decision science function. His team is responsible for the development of operations research, machine learning, artificial intelligence, and advanced analytical capabilities in the areas of network, facility, fleet, routing, and logistics strategy and optimization. He previously held technology, engineering & analytics leadership roles enabling revenue growth and operational efficiencies for multiple industries. Hema received his undergraduate degree in civil engineering from Osmania University India, MS degree in environmental engineering from The University of Memphis and MBA with supply chain & finance focus from University of Houston. Hema is a co-inventor on seven patents.
Related Episodes
Episode Transcript
Ashley K:
Welcome to the latest in our special series of Resoundingly Human podcasts, highlighting the finalist teams for the 2025 Franz Edelman Award. The Nobel Prize of Analytics, which will be awarded at the upcoming 2025 INFORMS Analytics plus conference in Indianapolis this April. These finalist projects represent teams from around the world who have leveraged advanced analytics to transform their organizations, address their most significant challenges and better serve their customers and communities. Today I’m joined by members of the team representing WM to discuss their finalist project in the lead up to the Franz Edelman competition. To start, I’d love to have you each introduce yourselves and your role in the finalist project you’re being recognized for in this year’s fund’s A Limit Award competition.
Marcel Dalby:
Hey, Ashley, good morning. My name’s Marcel Dalby. I’m the Vice President of Collection operations and Business Optimization here at wm. First off, I want to thank you for having us on the podcast of Resound Human. We’re happy to be here. We’re very excited to talk about our project, which we call Dynamic Route Optimization. Very excited that INFORMS has recognized the project for the prestigious Friends Edelman Award. Before we get into that, I would just say if you’re not familiar with wm, we’re formerly known as Waste Management. Many of you probably know us as the big green trucks that you see servicing your neighborhoods and around your cities providing waste and recycling needs, but we’re much more than that. We’re the North America’s largest waste recycling company and the leader in sustainability services. In terms of this project, I’m the business owner for the development of the operational side of the technology and the corporate leader for the teams of routers and dispatchers that ultimately use the technology that we designed and implemented.
Hema Pillutla:
Morning Ashley. My name is Emma Pula. I’m the senior director of decision sciences here at Waste Management in the wm in the business optimization group. In my role, I lead a group of OR and data science professionals in this group. So as part of this project, my team has led the analytics or algorithms and automation engines that we submitted as part of the transit settlement price.
Ashley K:
I’d love to hear how did collaboration between different teams or departments contribute to the success of your project?
Marcel Dalby:
Actually, I’ll start. I tell you that the collaboration between our teams was really the differentiator and really making this work. I’ll tell you that at wm, we’ve actually tried this twice over the last 15 years to use data and analytics to dynamically route or actually our industrial line of business, and we weren’t successful, frankly, the collaboration of our teams come together to dynamically optimize 22,000 customer services across 500 hauling sites and 4,000 routes a day was a herculean effort. If you think about getting all of the data that has to happen and has to be correct behind that, that’s no small task. So building an optimizer that can handle that much data and produce 4,000 routes and 45 minutes every night for tomorrow took a lot from our team of the operations research team and data and analytics. That’s really where him and his team will come in and I’ll let him talk about all the smart stuff there. But beyond that, really getting our field team to trust the optimization, really working with them hand in hand to test the solution and let them see it before it went live is really what really helped in that collaboration to get all of the field roles involved, even all the way down to our drivers to get them involved, get their feedback, and we can talk a little bit more about how we did that.
Hema Pillutla:
Yeah, this was a huge team effort. There were hundreds of team members in our corporate areas, the multiple functional areas, the business who came together, and we should remember this came about after the pandemic and most people were working remotely, both in North America and India. And it took a little bit of an effort for us to get there, a lot of passion from all the team members. And what helped also was the fact that we kind embrace agile technologies and standups and so forth as part of the project and kind of helped us bring the collaboration together much more tightly.
Ashley K:
What were some of the biggest hurdles your team faced when tackling this problem and ultimately, how did you overcome them?
Marcel Dalby:
Actually, I’ll highlight two kind of big hurdles that we had. The first being data integrity and then really earning trust in the solution with our field team. So in terms of data integrity, I just mentioned how many stops we route every night and we have to do it in 45 minutes, or we try to get it done in 45 minutes to make sure it’s ready for tomorrow. And if you think about our roll-off services, these are the big industrial boxes that we set off at a customer’s location or maybe a big compactor that would be at a hotel or shopping mall and some of those things. And it’s pretty dynamic. Some of those services are certainly on schedule, but many of them are on call where the contractor will actually call and say, Hey, I need four halls tomorrow, whatever it is. And because of that dynamic nature is really why we went after this project to route it.
Prior to this time, this was getting routed manually by dispatchers and routers in the field that would spend three or four hours every afternoon trying to put together the routes for tomorrow. And so there’s so many parameters. We knew that a human router just physically can’t assess all of those parameters and really optimize it. And so the biggest thing was getting the data right. Each of those individual services has 72 unique data points that has to be right. And I’ll let Hema talk about the technical side of the algorithm and how we tackled that. But getting that data and then keeping it right was a huge step for us and that’s really why we weren’t successful the first two times we tried this, but I’ll touch on kind of earning trust in the solution. So as we started this and we have 500 hauling locations across the United States and Canada, and as we started and plan to go live at each one of those 500, we would do what we called a conference room pilot where we would literally run the routes to the optimizer while the human router was building them for tomorrow, and they’d ultimately run the human generated routes.
But that router that’s very familiar with those stops and that route manager would look at the stops, look at the optimized solution and start to understand what it did, what halls did it have for tomorrow, what was in the mix, and then how did it work, what did it do that I didn’t do? And they could start to see some good ideas, if you will, that the engine or the optimizer would create that they maybe have never thought of. And really for us, it’s about optimizing those legs. If you’re familiar with logistics, it’s those deadhead miles where we are going to fetch a box to deliver it or removing a box and having to drop it back to our can yard. Those are just non-productive miles. So it’s really about how do we eliminate those? And the optimizer is really good at that. It can analyze all the parameters that a human just can’t. So I’ll stop there. I’ll let him talk a little bit more about the technical side of the hurdles.
Hema Pillutla:
Yeah, yeah, I think look, one of the biggest hurdles is, Marc alluded to it, we tried twice before and we were not successful, and there was a belief somehow that we can solve this routing problem through or and analytics. And as we reflected on those learnings from before, there are a couple of takeaways for us. One is we didn’t focus on data before, and I think Marcel talked about how we were ate this time around. The second thing is around sitting of the business and really understand the intricacies of the business needs. A lot of times we don’t focus on that piece of it, the details. We really spend time with our business stakeholder to get to the complexities of what goes on when somebody routes it, and lastly, it’s the engaging the business. So we learn from those three things, and fortunately this time around, we arranged as a joint products come team, so that made the collaboration more tighter and we were itrate in our approach to delivering it, and that’s really the magic that solved this Theron.
Ashley K:
Ultimately, how did your team balance the technical complexity of the solution while still ensuring the solution was practical and implementable?
Marcel Dalby:
Yeah, so I’ll leave it to Hammer to talk about the technical complexity, but I would just say a couple of things here as far as those conference room pilots that I mentioned. Those were critical, right? For the routers and the managers to see the solution before they had to execute it and be able to understand what it was doing, how the engine was working, and see the sort of good decisions that it would line up. In our business, we try to do what we call hall matching. So we try to make sure that the way you end this stop prepares you properly for the next stop. If you’re empty rails, you need the next customer to require you to the empty rails, not have a box on the back. If you have a box on the back of the truck, you try to line it up so that the next service needs that size box and it eliminates those non-productive miles, if you will.
So once the routers and route managers we’re able to see those solutions, they kind of got it right. They could see, Hey, this is better. And we actually could see in our analytics, Hey, this is a 10 hour route, and the optimizer was able to make it a nine and a half hour route, and that gives you enough capacity to actually do one more haul for the day with that driver. So that really helped drive trust in the optimization. The other thing that we did, and we still use it today, is we installed a digital feedback button for our drivers. So our drivers have a tablet in their truck and we actually put a button in it. The driver could push a button and give us feedback. Hey, this stop feels like it was out of place. You need to take a look. And often what we find is the feedback’s really good from our drivers, and if they see something that doesn’t quite make sense, it’s probably a data opportunity for us. One of those 72 data parameters might be wrong. And so it kind of put the stop somewhere that didn’t make the best sense. So that’s really helped us build trust but also enhance, if you will, the solutions that we’re building every day.
Hema Pillutla:
Yeah, look, we’re fortunate to have a really strong group of extremely competent or folks who have solved these kind of problems before, specifically our network optimization and route optimization in multiple lines of business at WM as well as in other industries. So that was a good thing for us to have. A second part of that is really, and these folks are used to the mathematical programming techniques, heuristics and meta heuristics and so forth. We knew right off the bat what technique to use for this problem. The second thing really we were fortunate to have is a strong engineering team who can actually build enterprise, get software products such skills to the company of the size of wm. So with that experience and background, really the most important part for us was to sit with the business folks and get to know what goes into how a human does the work and making sure all the intricacies are baked into our algorithms and beyond that, really the question becomes how do we scale this to the size of the company, which kind of took us multiple ations to tune our algorithms and to be able to build 4,000 routes under 45 minutes with close to 99.9% accuracy.
So that was, I try process is the most challenging piece of it. Beyond the rest of the data and so forth that Marshall talked about,
Ashley K:
I’d love to have you share your perspective on how you see analytics continuing to impact your industry over the next five to 10 years, and how do you hope to continue to explore analytics applications? So
Marcel Dalby:
We’re really excited about how we see analytics continuing to impact our business over the next few years. We really feel like the dynamic route optimization solution we implemented is just a start for us. We’re already looking at how we can use this in other collection lines of business. So we have industrial, commercial, and residential services and try to take those logistics strategies to those other lines of business, but we’re also seeing some kind of transportability in the way we’re doing the optimization. So if you think about our vehicle maintenance, our trucks are very maintenance intensive. They’ve got a chassis that has an engine, whether it’s diesel or CNG, but they also have a body that is operated by hydraulics for lifting the containers and compacting the waste to recyclable. So the equipment is very maintenance heavy, if you will, very maintenance intensive. So we’re using an AI driven optimizer to help us plan and schedule our maintenance work across a 14 day time horizon.
It helps us ensure that parts are available and in stock when we need them for that work. It helps us to marry up each repair to the most qualified technician that knows how to do that task. It helps us group jobs on any individual truck so that every time we do some repair on the truck, we don’t just do one job, we don’t just change the oil, we may change the oil and also do the brake job that maybe do two or three weeks from now. So it helps us group those together and really optimize the performance of our fleet and our technician assets. So seeing some nice results in our early pilots, that program as well.
Hema Pillutla:
Yeah, look, I think there has been explosion of data being collected in our industry for the past few years, and this data is coming from our trucks, the machines that we operate in, our recycling facilities, the operational technology we have at landfills. Really the question becomes how do you use the data combined with any other directional data sources to provide a meaningful insights analytics to the business that present a huge opportunity From a tech perspective, things are also evolving with lines being blurred, being analytics, the traditional or and new stuff like AI and a GI coming into place. So part of the job for the teams is to get ready and be current with the skills. That’s what I see as an opportunity. And then what it means to us though, more important than ever is to drown the noise and make sure that we pursue meaningful opportunities that really drive impact for our company and our customers. And really what it means to us then is specifically for me and my group, is to make sure we combine the strategic aspects of it, the planning, execution, and performance to combine for the business end-to-end insights of the business. Another piece of it is we have some emerging areas of the company, like our push collections where we have an opportunity for us to make a difference with all the data we collect.
Ashley K:
And finally, what advice would you give to teams just starting out on their analytics journey, especially those who might be aiming for the Franz Edelman Award someday?
Marcel Dalby:
So again, I’ll let Hema talk about the technical side of the business and kind of the analytical solutions. But from the business side, I’d probably say two things. Data quality and change management were big for us as we know in any technical solution, right? If data’s not good, it’s kind of garbage in, garbage out. Now that phrase means a little something different for us here at wm, but you get my point. Data quality is more critical than ever. As companies try to divide, drive analytical solutions and optimizers, and especially as we use AI to better interact with our operators and with our customers. The second piece of advice I’d give is focus on the change management. Over the last two years, I found myself saying, at wm, the technology alone will not solve our problems. So we have to have solid communications, training, process adoption, accountability to those processes to ensure everyone in the organization understands what’s going on and what’s changing and how that impacts our role. So we created trainings not just for the routers that actually use the tool, but for our drivers, for our managers. We talk a lot with our senior leaders about how the tool’s working and what’s it producing and how are we seeing it drive gains for our business. So I really think those two things are what’s made a difference for us.
Hema Pillutla:
Look, as a person straddling technology analytics, I’m really passionate about business impact. My advice to the analytics folks would be to know the company and focus on the problems that the companies care about that your company cares about. And I think also as analytics professional for me, it’s not to be enamored or biased towards a particular analytical technique or approach, but using the right tool in the toolbox for the problem at hand. The second one I would say is to take the art of possible and show to your stakeholders in the company that you’re working on, partner with them and make sure that analytics can make a difference to the problems that they care about. And then lastly, for those folks who are aiming for France or women, I would say go for it. I think for folks who are in the practice area, this is the greatest honor for all the analytics professionals. And I think being inspired by attending in forms conferences, learning from the previous winners or the finalists would help the analytics folks. And really from our own experience, my own inspiration to apply for the France Alman came after we attended the Phoenix Conference close to two years back, and then the support of the organization, we went ahead. It is a lot of hard work, I would say, but it’s worth for the company and the team members that you represent, showcasing how analytics and or can improve companies and drive impact.
Ashley K:
Thank you both so much for taking the time to share this special insight into your finalist project. I wish you the very best of luck in the Franz Edelman Award competition, and I look forward to meeting you and the rest of your team in Indianapolis for the 2025 Forums Analytics Plus conference.
Marcel Dalby:
Thanks, Ashley.
Ashley K:
If you’d like to learn more about today’s episode and guest, visit resoundingly human.com and check out our show notes. The podcast is also available for streaming and download on Amazon Music, apple Podcasts, Google Podcasts, and Spotify. Wherever you listen, please be sure to leave a five star review to help others find and enjoy the podcast. Until next time, I’m Ashley K and this is Resoundingly Human.