Published: March 29, 2022
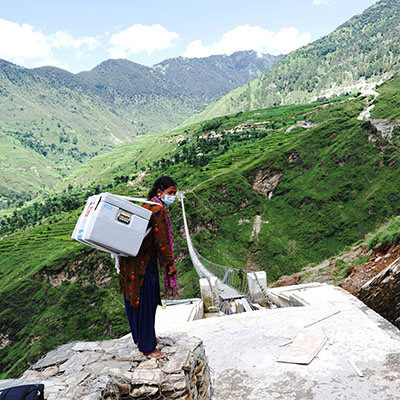
This podcast is part of a special series featuring the 2022 finalist teams for the INFORMS Franz Edelman Award for Achievement in Advanced Analytics, Operations Research and Management Science, the most prestigious award for achievement in the practice of O.R. and advanced analytics.
For more than four decades, the Edelman Award has recognized contributions that are transforming how we approach some of the world’s most complex problems. Finalists for the Edelman Award have contributed to a cumulative impact of more than $336 billion since the award’s inception, as well as countless other nonmonetary benefits. The winner of this year’s award will be announced at the 2022 INFORMS Business Analytics Conference, April 3-5.
Joining me for this episode are Dimitris Bertsimas, professor with the MIT Sloan School of Management and Najat Khan, Chief Data Science Officer and Global Head, R&D Strategy & Operations with Janssen Research & Development, LLC, to discuss the finalist entry from the team at Janssen Pharmaceutical Companies of Johnson & Johnson (Janssen).
To accelerate the development of the Johnson & Johnson COVID-19 vaccine, the R&D Data Science team at Janssen worked with the Massachusetts Institute of Technology (MIT) to co-develop and refine a machine learning-based COVID-19 epidemiological disease spread model, building on MIT’s DELPHI scenario analysis tool, capable of predicting future COVID-19 infection spread months in advance at a global level. This model enabled Johnson & Johnson to expedite a highly accurate clinical trial for the first single-dose COVID-19 vaccine, as well as gain valuable insight on virus variants.
One of the big questions from a data science innovation perspective was, can we predict the considered unpredictable, which was where would COVID-19 be spreading many months down the line. And why is this important? If we can predict the right hot spots where COVID-19 is, then we can actually enhance the data we collect, and also accelerate phase 3 trials. Why is that important? Because we can get our vaccines to patients and people earlier. Like I say, in a pandemic, every day counts.
Interviewed this episode:
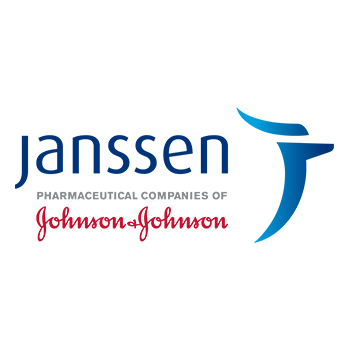
Najat Khan, Dimitris Bertsimas
Janssen Research & Development, MIT
Najat Khan, PhD, is the Global Head (Vice President) for Strategy & Operations at Janssen Research & Development. In this role, Najat shapes the R&D strategic vision and operationalizes across multiple high priority areas to ensure Janssen R&D continues to deliver on transformational medicines for patients. These include building new capabilities such as creating a best-in-class R&D data science organization by selecting the highest priority questions to pursue, systematically deploying Data Science capabilities, while being a key catalyst in forming strategic external partnerships to accelerate on-going efforts. Najat also collaborates with the various Janssen R&D therapeutic and functional heads, as well as Commercial and Business Development leaders on a wide variety of cross-functional strategic, portfolio related, and operational priorities while ensuring the respective insights are incorporated effectively within the broader Janssen community.
Prior to her current role, Najat was the Head of R&D Strategic Initiatives. In a brief period, she was able to develop and execute a comprehensive program comprising of 25+ of Janssen’s R&D strategic initiatives, and was instrumental in launching our data science efforts, defining our R&D strategy for pursuing opportunities in new diseases area, enhancing early stage external innovation, etc.
Najat has more than 10 years of global healthcare experience in pharmaceutical, biotechnology, business and academia. Prior to joining Janssen, she was a Principal at The Boston Consulting Group (BCG) where she has led and executed multiple strategic initiatives across research, commercial, business and clinical development, mergers and acquisitions and operations. Within R&D, areas of focus included portfolio governance and decision-making, R&D operating model optimization (including both clinical development and operations), external innovation and Digital Health. While at BCG, Najat also led the implementation of the Women’s Mentorship program and recruitment and retention efforts as recruiting director and career development advisor.
Najat received her BA in Chemistry with a minor in Economics from Colgate University and her PhD in Organic Chemistry from the University of Pennsylvania with publications in high impact journals such as Nature, Proceeding of the National Academy of Sciences, etc. She was also a fellow at the Penn Center for Innovation and led multiple licensee / partnership arrangements between UPenn and leading pharmaceutical and large cap biotech companies.
Dimitris Bertsimas is the Boeing Leaders for Global Operations Professor of Management, a Professor of Operations Research, and the Associate Dean for the Master of Business Analytics at MIT.
A faculty member since 1988, his research interests include optimization, stochastic systems, machine learning, and their application. In recent years, he has worked in robust optimization, statistics, healthcare, transportation and finance. Bertsimas was a cofounder of Dynamic Ideas, LLC, which developed portfolio management tools for asset management. In 2002, the assets of Dynamic Ideas were sold to American Express. He is also the founder of Dynamic Ideas Press, a publisher of scientific books, the cofounder of Benefits Science, a company that designs health care plans for companies, of Dynamic Ideas Financial, a company that provides financial advice to customers, of Alpha Dynamics, an asset management company, P2 Analytics, an analytics consulting company and of MyA health, a personalized health care advice company.
Bertsimas has coauthored more than 200 scientific papers and the following books: Introduction to Linear Optimization (with J. Tsitsiklis, Athena Scientific and Dynamic Ideas, 2008); Data, Models, and Decisions (with R. Freund, Dynamic Ideas, 2004); Optimization over Integers (with R. Weismantel, Dynamic Ideas, 2005); and The Analytics Edge (with A. O’Hair andW. Pulleyblank, Dynamic Ideas, 2016). He is former department editor of Optimization for Management Science and of Operations Research in Financial Engineering. Bertsimas has supervised 59 doctoral and 31 Master students. He is currently supervising 22 doctoral students. A member of the National Academy of Engineering and an INFORMS fellow, he has received numerous research awards, including the Harold Larnder Prize (2016), the Philip Morse Lecturship prize (2013), the William Pierskalla best paper award in health care (2013), best paper award in Trapsoration (2013), the Farkas Prize (2008), the Erlang Prize (1996), the SIAM Prize in Optimization (1996), the Bodossaki Prize (1998), and the Presidential Young Investigator Award (1991–1996). He has also received recognition for his educational contributions: The Jamieson prize (2013) and the Samuel M. Seegal prize (1999).
Bertsimas holds a BS in electrical engineering and computer science from the National Technical University of Athens, Greece, as well as an MS in operations research and a PhD in applied mathematics and operations research from MIT.
Episode Transcript
Ashley Kilgore:
This podcast is part of a special series featuring the 2022 finalist teams for the INFORMS Franz Edelman Award for Achievement in Advanced Analytics, Operations Research, and Management Science, the most prestigious award for achievement in the practice of OR and advanced analytics. For more than four decades, the Edelman Award has recognized contributions that are transforming how we approach some of the world’s most complex problems. Finalists for the Edelman award have contributed to a cumulative impact more than 336 billion since the award’s inception, as well as countless other non monetary benefits.
Ashley Kilgore:
The winner of this year’s award will be announced at the 2022 INFORMS Business Analytics conference held April 3rd to the fifth in Houston, Texas.
Ashley Kilgore:
Joining me for this episode are Dimitris Bertsimas professor with the MIT Sloan School of Management and Najat Khan, chief data science officer and global head of R&D strategy and operations with Janssen Research and Development LLC, to discuss the finalist entry from the team at Janssen Pharmaceutical companies of Johnson and Johnson. To accelerate the development of the Johnson and Johnson COVID-19 vaccine, the R&D data science team at Janssen worked with the Massachusetts Institute of Technology to co-develop and refine a machine learning based COVID-19 epidemiological disease spread model building on MIT’s Delphi scenario analysis tool, capable of predicting future COVID-19 infection spread months in advance at a global level. This model enabled Johnson and Johnson to expedite a highly accurate clinical trial for the first single dose COVID-19 vaccine, as well as gain valuable insight on virus variants.
Ashley Kilgore:
Thank you both so much for joining me. I’m looking forward to discussing Janssen’s work.
Najat Khan:
Thanks for having us, Ashley.
Dimitris Bertsimas:
Thanks, Ashley.
Ashley Kilgore:
Looking back over the pandemic timeline, when and how did development of the Johnson and Johnson COVID-19 vaccine begin to take shape?
Najat Khan:
When the pandemic hit, all of J and J, we really went into action in terms of how can we develop an effective and safe vaccine. We looked at what are the hardest problems for us to solve that could get something that’s really beneficial for people around the world? We used different scientific innovations. We used data science innovations. One of the big questions from a data science innovation perspective was, can we predict the considered unpredictable, which is where would COVID-19 be spreading many months down the line? And why is this important? If we can predict the right hotpots where COVID-19 is, then we can actually enhance the data we collect and also accelerate phase three trials. Why is that important? Because we can get our vaccines to patients and people earlier. Like I say, in a pandemic everyday counts. So we started on this journey in March 2020 as soon the SARS COVID two was sequenced and partnered with Dr. Dimitris Bertsimas at MIT in order to develop machine learning models that helped us solve some of the most challenging problems for us to solve.
Ashley Kilgore:
Now with multiple vaccine options in development simultaneously, what was unique about Janssen’s approach?
Najat Khan:
I think Janssen was really forward thinking in terms of the use of data science to accelerate and enhance our development. So let me just give you an example. As I mentioned, being able to predict where the COVID hotspots are going to be many months down the road, I mean, imagine this in March 2020 was a challenging problem. There was such little information that we had to begin with. So what we did was we partnered with MIT to develop machine learning models that could forecast several months in ahead where these hotspots were going to be, not just at a country level, but at a province county level. So great resolution and precision in our predictions. And the other aspect it also did was the models were trained to be able to predict the government interventions and social compliance. This was a very unique signature that’s only true for this pandemic.
Najat Khan:
So that’s why it took a lot of ingenuity in order to develop these models, test them to ensure they’re accurate, and then to deploy them. This model was critical and instrumental to our decision as to which countries we went to and what specific locations we went to for our phase three trial. And I’m happy to share we had over 90% accuracy in the hotspots that were predicted by this joint Janssen MIT model, machine learning model. Second, given the predictions were so good, we were able to shave the time from our development timeline by about eight weeks. And as I mentioned before, every moment, every day counts in a pandemic. Number three, it actually allowed us to have a very global trial by being completely unbiased and going where the data was telling us to go, where the disease would be.
Najat Khan:
And so we ended up with a global trial launched in the US, but also South Africa, Brazil, and many other countries. The fourth, which was very exciting to work with Dimitris on is we actually customized our model to over index predictions in areas where there’s high diversity. This resulted us in having a high diverse trial. And that’s important because we want our data, clinical data to be representative of those that we would vaccinate in the world.
Najat Khan:
And the fifth and last point, is it also by following the data in an unbiased fashion, we were able to go to regions where there are variants due to high incidents of COVID-19 that also helped us get really important scientific data on the different variants and how vaccines would hold up against them.
Ashley Kilgore:
Could you highlight some of the other challenges associated with the development of the Johnson and Johnson COVID-19 vaccine?
Najat Khan:
Yeah, I’m happy to touch on some of the challenges in terms from a data science perspective. The example I just mentioned, first is getting global data. All data quality across the world is not the same or it’s not standardized. So I remember Dimitris and I, our teams worked tirelessly. We would have joint meetings every day at 7:00 PM. Dimitris, if you remember, to actually clean the data, to get a lot of information boots on the ground as to what’s happening in the different countries, to then be able to train the models appropriately. Your models are only as good as the data and your assumptions that you’re putting in. The second thing is when we… The second complexity was we started these modeling efforts in May of 2020. We only had two months of the pandemic before that. So we didn’t really have a lot of retrospective or historical data, which meant that being able to develop models that can do scenario based modeling was extremely important.
Najat Khan:
And this is where Dimitris’ and the MIT’s model was exceptional, so that we could really be flexible in terms of our recommendations and fine tune it as we learned more with every month that went by. And maybe the third thing I would say is machine learning data science is upending, how we do things in R&D across the industry. And that comes with change. And that requires changing hearts and mind, which is the right thing to do. But of course there’s challenges and it’s a journey to be able to do that. And I’m excited to see that we are actually able to do that and take these calculated risks to really advance our medicines and vaccines.
Ashley Kilgore:
Could you share more details on the model your team developed to help circumvent these challenges you mentioned?
Dimitris Bertsimas:
So the model we developed, the initial model is of the classical SEIR variety. We have a susceptible population, infected population, exposed population. And we build nominal differential equations between these quantities that predict the evolution of the disease. An important component, which was one of the most significant development is that we took into account the fact that government interventions are significant and how people behaviorally react to these interventions. For example, the behavior of people in Lima, Peru, and people in let’s say in New York City or in Florida is quite different of how people adapt to these interventions. And we had a mathematical way of modeling how various populations react to these government interventions. The combination of therefore, more of capturing that as well as the more elaborate modeling of the various states of the people, for example, hospitalized, recovered, and so forth. Machining modeling led to overall more accurate predictions.
Dimitris Bertsimas:
And the fundamental idea of what we did is that we used the models, used parameters. We selected parameters so that the data we have up to that point, the closeness between prediction and reality was as close as possible. And of course this meant different parameters for different areas of the world.
Najat Khan:
If I can just build on one thing that Dimitris said, when we started partnering with Dimitris, there were so many models that were available. But what I really liked about the work that MIT had done is the level of rigor and validation that Dimitris had put into it, in addition to these different scenarios and sort of the dynamic approach to the model. That was extremely important. And that’s something that we continue together as a team. And I think that’s why you have the impact that you see. It’s not just about the complexity of the model, but actually the fact that we had an iterative approach to refine and enhance the model as we learned more with every day and every week that went by. So just wanted to highlight that, which is an extremely important part of how we do data science to be totally integrated in the development of the medicine versus just using output that comes in here and now. It’s core part and parcel of our work.
Ashley Kilgore:
And ultimately, how do the implementation of this model into the development of the vaccine impact both its testing and approval process?
Najat Khan:
First of all, when we do phase three trials, we had to select which countries should we go to. And then we have to select which exact locations within that country we go to set up the sites. And like I said before, this model was instrumental in terms of the countries. We would’ve gone very differently if we did not use the model here and also the sites that we selected. And we tracked and monitored how we did from an incidence perspective. And that’s why we know that there’s 90% accuracy in the predictions from our model.
Najat Khan:
The other piece you mentioned the approval being six to eight weeks earlier ahead of time, ahead of schedule was a huge deal for us because… And that was one of the big factors was because we were in the right place at the right time to run and design and conduct our trial.
Najat Khan:
The other thing, and these are intangibles but highly important, having clinical data on variants. There were variants in Brazil, variants in South Africa. We were right in the middle when the first variant surge happened in South Africa. Also, having a highly diverse trial was extremely important to us, both for this vaccine, but it also showed us a way to do things differently for the rest of our pipeline and portfolio. So this is not a one off. This is actually the learnings here. We’re leveraging for the entirety of our pipeline and portfolio. So that was extremely important learning for us as well. And the work continues. The team is continuing to use data science, world evidence to really understand the effectiveness of the vaccines in the real world, especially with these new variants that are coming up.
Ashley Kilgore:
I’d like to thank you both again for joining me and would like to wish you and the rest of your team, the best of luck in the 2022 Franz Edelman competition. Are there any final thoughts you’d like to share regarding Janssen’s finalist project?
Dimitris Bertsimas:
Well, something that I would like to mention is the joint effort and the hard work by a sizable team from both parties. I remember that from let’s say May until to the day, in the beginning, we were meeting daily five to seven days a week, every day. And it was really this very close collaboration that made it feasible for the model to be helpful for the vaccine effort. It is something that will stay in my career for many years. I’m certain.
Najat Khan:
Maybe one thing I’d like to add is over the last few years, people have hypothesized as to how you can completely transform discovery development of medicines, vaccines using data science. This is one of the clearest examples of doing that for one of the highest priority programs. The stakes were so high. And I just want to thank both the teams, but then also just overall the leaders for taking the bet on data science and having the will and the fortitude to make decisions in a very unbiased and completely data driven way.
Najat Khan:
Because as we’ve seen from the impact, it helps patients. And that’s why we do what we do, but I can’t underscore that enough because that is the only way, no matter how many advances and innovations you have, that will be implemented and applied and have impact for overall outcome. So I think this is one of the first big, great examples of that. And I see much more to come. The potential is so high. So I want to thank Dimitris for being such a close partner. Clinical trial development was not Dimitris’s forte, but this is what shows you the combination of different disciplines in the integration to solve new problems. We can actually do things better. And that’s why the great operational research that Dimitris does coupled with everything that we do in clinical development and discovery, together we can be stronger and better for our patients that we serve.
Ashley Kilgore:
Want to learn more? Visit resoundingly human.com for additional information on this week’s episode and guest. The podcast is also available for download or streaming from Apple podcasts, Google play, Stitcher, and Spotify. Wherever you listen, if you enjoy Resoundingly Human, please be sure to leave a review, to help spread the word about the podcast. Until next time, I’m Ashley Kilgore. And this is Resoundingly Human.
Want to learn more? Check out the additional resources and links listed below for more information about what was discussed in the episode.
2022 INFORMS Business Analytics Conference, April 3-5, Houston, TX